Artificial Intelligence in Management
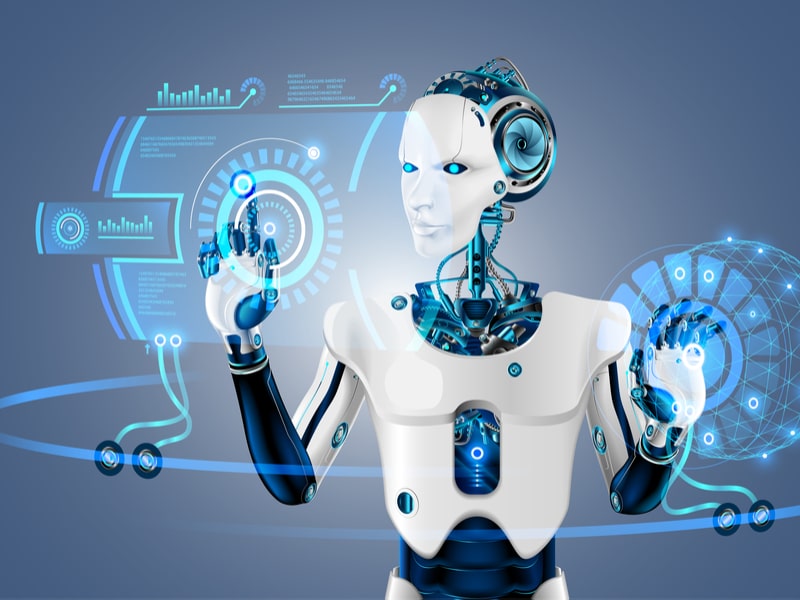
Much has been said about cognitive computing, which is considered the current generation of AI. In other words, learning by systems came to succeed the stage of very fast inputs and outputs by machines. This means that new information can be generated from the data with which the systems were fed.
As it happens, there has been little concrete evidence so far, even though the IT industry has been talking about neural networks for decades. However, according to this IBM story, the time is now, because both hardware and software are ready for artificial intelligence.
Cognitive computing is really the ball of the day
There’s no doubt computers are learning. After all, back in the early days, processors were able to perform calculations; then they evolved to the use of programmable systems (as we know today), and, as we have seen, are now able to process information based on learning from previous experiences. It is a similar operation to our brain, since we are receiving information and processing it to acquire learning.
Artificial Intelligence, the software reads the patient’s information and sends alerts to the medical team if the patient’s condition is, for example, of generalized infection.
However, when it comes to artificial intelligence in management, there are some limitations. Machines are more “trained” than “programmed”. Because of this, the various processes generally require immense amounts of labeled data to perform complex tasks accurately.

Let’s learn more about three of these limitations, and the possible solutions that are emerging to overcome them:
Data Cataloging
This is one of the major obstacles imposed by the AI in the path of companies: to carry out the cataloguing of this data. Obtaining it can be very difficult, or even impossible.
This is because most AI models are trained through “supervised learning,” which means that we humans need to catalog and categorize the underlying data. For example, companies that develop self-drive cars are hiring hundreds of people to manually record prototype hours and video information to help train the vehicles.
On the other hand, new techniques are emerging to optimize this tremendous work. One is the in-stream supervision, recently presented. Through which data can be catalogued during the course of natural use of a prototype.
Obtaining large data sets for training
The current machine learning moment requires not only catalogued data, but an immense amount of it. A deep learning process requires thousands and thousands of records for models to satisfactorily perform their complex recording tasks and, in some cases, millions of them to perform at the same level as a human being.
This is another important limitation, since accessing this amount of data can be a very difficult task. And each minimal variation in an assigned task may require a new amount of data to continue learning.
The problem of “explainability
“Explainability”: even if the term seems new, it has haunted AI systems for some time. But it has become a more troubling issue as deep learning processes have evolved. Larger and more complex complexities make it more difficult to explain, in human terms, why a certain decision was made.
This is one of the reasons why the adoption of some tools and AI is restricted in areas where the need to explain is useful, or even required. In addition, as the application of artificial intelligence in management expands, regulation could also leverage the need for more “explainable” models.
Artificial intelligence in management: start now!
- Get to know and become familiar with AI: take your time to learn the basics and what resources might be useful to you;
- Identify what problems you want to solve with AI: now that you know the basics, think about where you can apply them and how this can optimize or improve your processes;
- Think concrete: for AI innovations, the sky is the limit. However, you need to pay attention to the available budget and whether the investment will bring value to your business;
- Know your bottlenecks: there is a big difference between what you want to achieve and what the team can do. Take this into consideration before implementing the IA;
- Create a pilot project: once you have mapped your company’s areas, start defining a plan. If you want, you can hire an expert or a consultant;
- Create a task force to integrate data: organize the collection and cross-referencing of data to prevent unnecessary information from hindering your view of what’s important;
- Start small: choose an area or a company process to start with. Then expand;
- Include storage as part of your plan: because of the large volume of data generated, it is important to know where and how you will store it so you don’t lose anything and ensure information security;
- Incorporate IA into your daily tasks: some employees may become fearful about the technology. In order for them to see the benefits, it is important that IA is part of their daily lives;
- Be balanced!
In addition, the tool provides you with data on deliveries and performance, reducing your time invested in micro-management and increasing your availability to think about strategic solutions for the team. And the best: you gain time to stay on top of the main trends in technology.